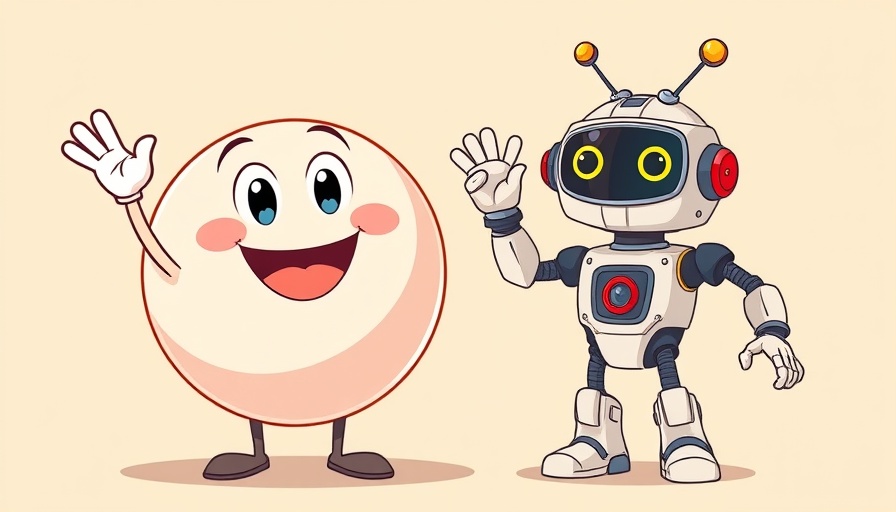
Revolutionizing AI Model Evaluations with Yourbench
In the rapidly evolving landscape of artificial intelligence, evaluating AI models has become a crucial task for enterprises. Today, standard benchmarking methods often fall short, as they measure general capabilities rather than specific performance for an organization’s unique needs. This is where Yourbench, a new tool from Hugging Face, steps in. It allows organizations to create custom evaluations using their own data, ensuring that model assessments are more relevant and actionable.
Yourbench: A Customized Approach
Launched by Hugging Face, Yourbench enables users to conduct personalized benchmarking tailored to specific tasks within their organizations. According to Sumuk Shashidhar from the evaluations research team, the tool supports custom benchmarking and synthetic data generation from any document, which is a significant advancement in model evaluation. It empowers companies to see how well models respond to tasks that matter to them.
The Process of Custom Evaluation
Yourbench streamlines evaluation through a three-step process: Document Ingestion, Semantic Chunking, and Question-and-Answer Generation. Document Ingestion addresses varying file formats, while Semantic Chunking focuses the model's attention by refining documents to fit context window limits. Once processed, questions are generated from the input data, allowing enterprises to assess potential models effectively.
Understanding Cost and Performance Trade-offs
While Yourbench exhibits impressive capabilities, it does demand significant computing resources. Shashidhar acknowledges this challenge and indicates that Hugging Face is actively expanding their computational capacity. With partnerships in place, such as with Google Cloud, Hugging Face aims to ensure that organizations can utilize Yourbench effectively without prohibitive costs.
Navigating the Limitations of Benchmarking
Despite the advantages of tools like Yourbench, some experts caution that benchmarks alone cannot capture the daily performance and safety of AI models accurately. Different methodologies, such as Google DeepMind’s FACTS Grounding which assesses factual accuracy, have emerged in response to the limitations of traditional benchmarks. This indicates a shift towards more nuanced evaluation processes that consider a model's practical utility in real-world applications.
Conclusion: A Call to Embrace Custom Evaluation Tools
For enterprises looking to leverage AI effectively, tools like Yourbench may well represent the future of model evaluation. By focusing on relevance and specificity, they provide insights that generic benchmarks cannot. To stay ahead in a competitive market, organizations must explore these innovative solutions to ensure their AI deployments are both effective and aligned with their unique operational goals.
Write A Comment