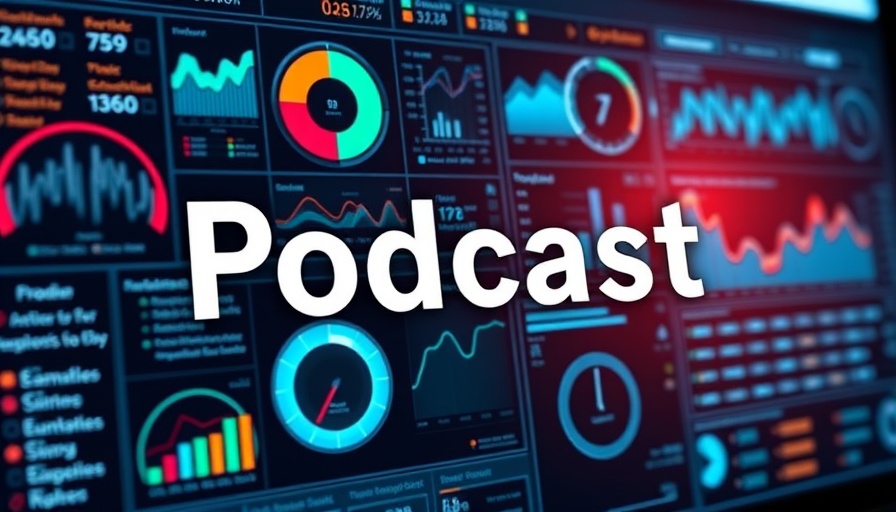
Understanding A/B Testing Pitfalls: Insights from Sumit Gupta
A/B testing has emerged as a cornerstone methodology in data-driven decision-making across various industries. As businesses strive to optimize their product features, understanding pitfalls becomes essential to improve accuracy and efficiency. Recently, we spoke with Sumit Gupta, a Business Intelligence Engineer at Notion, who shared invaluable insights on A/B testing, based on his extensive experience.
From Adversity to Expertise: Sumit Gupta's Inspiring Journey
Sumit Gupta's path to becoming a data science expert is nothing short of inspirational. Growing up in Dharavi, one of Mumbai’s largest slums, he discovered early on the transformative power of education. His journey from these challenging beginnings to major tech firms like Dropbox underscores the perseverance needed in the data field. As he remarked, “Hard work is irreplaceable; it’s about continuous upskilling.” This ethos ultimately led him to specialize in data science—where he now advises on best practices in A/B testing.
Common A/B Testing Mistakes: Gupta's Overview
In our conversation, Gupta highlighted two critical pitfalls typical of A/B testing:
- Biases in Experimentation: Teams often stop tests prematurely, resulting in unreliable data. Gupta pointed out that rushing can lead to false positives or negatives, stressing the need for established performance metrics that dictate when to terminate a test. “Don’t stop the test early; ensure you’re working with enough data,” he advised.
- Executive Pressure: He noted that executives may sometimes push teams for quick results based on insufficient data, which can compromise decision-making. “At Dropbox, even minor percentage changes in conversion rates could lead to millions in revenues. This emphasizes the importance of patience and thorough analysis,” Gupta explained.
The Nuances of A/B Testing in Marketing and SEO
Interestingly, A/B testing in the fields of SEO and marketing presents unique challenges, especially concerning Google’s policies against duplicate content. Unlike traditional product A/B testing, marketers opt for pre-post testing, a method where performance metrics are compared before and after changes are implemented.
“For effective SEO, we cluster similar pages to analyze performance shifts rather than running side-by-side versions of content,” stated Gupta. This adaptation showcases a shift in strategy that many marketers are beginning to embrace as they navigate the complex landscape of online visibility.
Broadening Perspectives on A/B Testing
The issues surrounding A/B testing are not isolated. The challenges discussed by Gupta resonate across many domains, suggesting a need for a more nuanced understanding of experimentation. According to several experts, including those covered in our referenced articles, A/B testing limitations can include:
- Sampling bias, which disrupts the representativeness of test populations.
- Confounding factors that obscure causal relationships during testing.
- Ethical issues that arise from conducting tests without user consent. Emphasizing the importance of fairness and respect in data practices is crucial.
Conclusion: The Need for Continuous Learning
As A/B testing becomes increasingly challenging yet essential, the onus is on data-driven professionals to refine their approach. Continuous education around emerging trends and statistical methods is vital. To thrive in the fast-paced digital economy, embracing a culture of learning, ethical practices, and rigorous testing will pave the way for better decision-making.
Write A Comment